Multimodal AI: Understanding Its Core Concepts and Applications

Multimodal AI is a cutting-edge field in artificial intelligence that aims to mimic the human brain's ability to process information from multiple senses simultaneously. Just as humans use their senses to experience the world, multimodal AI combines different types of data, such as images, video, audio, and text, to understand and interact with its environment more effectively.
At its core, multimodal AI involves the integration of various data types, or 'modalities'. For example, in the human context, if you see and taste a carrot, you can identify it quicker than if you were blindfolded. Similarly, multimodal AI combines different inputs to infer more accurate results. This method contrasts with standard AI, which typically focuses on a single task or modality, like image recognition or language processing. Multimodal AI can process two or more information streams, enhancing its ability to deduce what it's looking at.
The benefits of this approach are manifold. Multimodal AI tends to yield more accurate results compared to unimodal approaches, as it reduces the chances of misinterpreting data inputs. Essentially, the combination of multiple data types leads to a more comprehensive understanding, akin to a '1+1=3' scenario, where the whole is greater than the sum of its parts.
In practical terms, multimodal AI has diverse applications. For instance, in business, it can offer more intelligent insights into planning and decision-making. By analyzing data from various sources, multimodal AI can provide nuanced understandings of complex scenarios. This ability is particularly useful in predictive maintenance, where the AI, by taking inputs from multiple sensors, can make more informed decisions about the servicing needs of machinery.
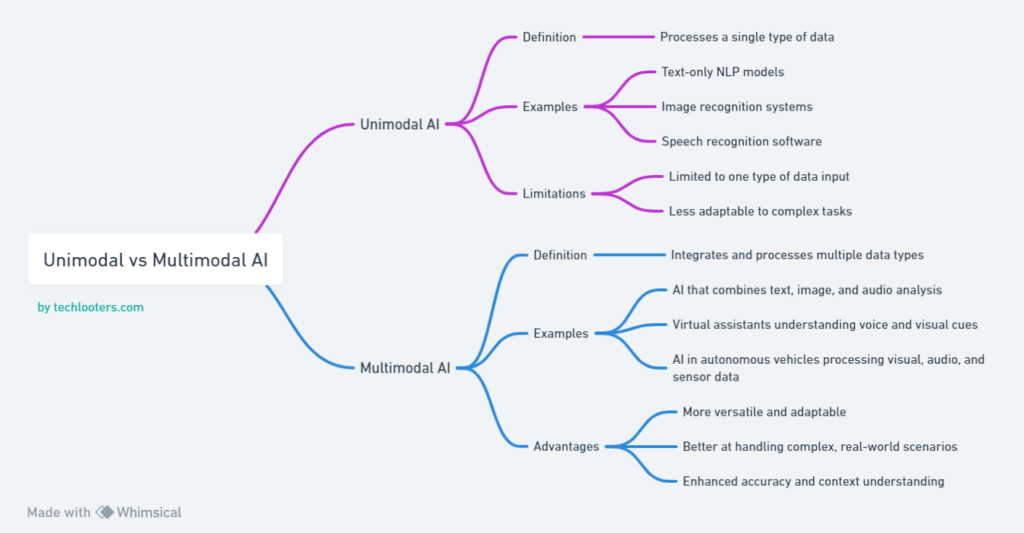
Another key area of multimodal AI is its application in deep learning. Multimodal deep learning, a subfield of machine learning, trains AI models to find relationships between different data types. This method is crucial for tasks like emotion recognition, where understanding human emotions requires analyzing both visual cues (like facial expressions) and auditory cues (like tone and pitch of voice). Such a holistic approach is essential for AI to match human intelligence in understanding and reasoning about the world.
Multimodal AI's future looks promising, with ongoing research tackling core challenges in data fusion, where information from different modalities is combined. Effective fusion is challenging due to the heterogeneous nature of multimodal data, but advancements in this area are crucial for the further development of multimodal AI technologies.
Overall, multimodal AI represents a significant leap towards creating AI systems that can perceive and understand the world in a way that is closer to human cognition. Its applications range from enhancing business insights to improving the accuracy of emotion recognition, making it a pivotal area of research and development in the field of AI.
Exploring Microsoft's Role in Advancing Multimodal AI Technology
Microsoft has been a key player in the realm of multimodal AI, a field at the intersection of multiple modalities like text, images, and other data types. This approach aligns with human perception and problem-solving, which leverage multiple senses. Multimodal AI systems are especially beneficial in areas like productivity, health care, creativity, and automation. They are built upon the fusion of vast datasets and advanced architectures like transformers, offering a more natural and seamless support compared to single-modality systems.
A significant focus for Microsoft has been the responsible development and deployment of these AI systems. The company has been building upon open-source models and studying cutting-edge models developed within Microsoft and by partners like OpenAI. The Aether Committee at Microsoft plays a crucial role in studying foundation models and new applications, with a focus on identifying potential risks and harms. These efforts are critical for planning mitigations and integrating these models into products responsibly.
Microsoft's research highlights various challenges and observations in multimodal AI
- Combining Different Content Types: The fusion of different modalities can lead to unintended harms. For instance, vision and language models may unintentionally reinforce societal biases. Studies have shown that image generation models like DALL-E v2 can sometimes produce outputs that lack diversity or reinforce stereotypes.
- Navigating Distributional Shifts and Spurious Correlations: Large and diverse internet-scale data doesn't always reflect real-world distributions. This can lead to models underperforming due to distribution shifts and spurious correlations. For example, Microsoft found that models like CLIP can be biased by coincidental features, being accurate in some scenarios but not in others.
- Decomposing Evaluation for Controllability and Precision: Evaluating multimodal AI involves assessing how well models capture important aspects of input prompts. Microsoft's research indicates that general-purpose scores like Fréchet inception distance (FID) are not sufficient for this. New protocols are required for a more comprehensive evaluation that accounts for aspects like spatial understanding.
- Leveraging Adaptation and Continual Learning Approaches: To address the gap between offline measures and real-world capabilities, Microsoft advocates for adaptation and continual learning approaches. This involves considering real-world variables like user feedback and unseen object categories. For example, mixed-reality systems have been developed for continual learning, allowing users to interactively provide labels and improve model performance over time.
Microsoft's involvement in multimodal AI is marked by a commitment to responsible AI practices, continuous innovation, and tackling complex challenges. This includes addressing biases, improving model accuracy and controllability, and adapting AI systems to real-world conditions, making Microsoft a significant contributor to the advancement of multimodal AI technology.
Deciphering the Meaning of Multimodal AI: A Comprehensive Overview
Multimodal AI represents a significant advancement in the field of artificial intelligence by transcending the limitations of traditional unimodal models. Unlike unimodal models, which focus on a single type of data, multimodal models integrate and process information from multiple sources, such as text, images, audio, and sensor data. This integration creates a unified representation of information, enabling a more nuanced and comprehensive understanding of the world, akin to human cognition.
Key Aspects of Multimodal AI
- Enhanced Accuracy and Performance: Multimodal models outperform unimodal models by integrating multiple perspectives, leading to better accuracy and performance in various tasks.
- Richer Context and Understanding: By analyzing data from multiple sources, these models gain a deeper understanding of the context and meaning of information, thereby offering a more holistic view.
- Robustness and Generalizability: Multimodal models are less prone to errors and noise in individual modalities, resulting in more robust and generalizable outcomes.
Applications and Use Cases
Multimodal AI has diverse applications, reflecting its growing importance:
- Text-Image Models: Used for image captioning, visual question answering, and image retrieval.
- Text-Audio Models: Applied in automatic speech recognition, sentiment analysis, and speaker recognition.
- Multimodal Attention Mechanisms: Enhance the focus on relevant information, capture complex relationships, and improve model interpretability.
Emerging Trends and Future Directions
The field of multimodal AI is evolving rapidly, with emerging trends including:
- Self-supervised Learning: Reduces the need for labeled data, making training more efficient.
- Multimodal Transformers: These powerful architectures process complex multimodal data, leading to performance breakthroughs.
- Explainability and Interpretability: Techniques are being developed to understand decision-making in multimodal models, enhancing trust and responsible AI practices.
A Multimodal Future
Multimodal models signify a leap forward in AI capabilities, indicating a future where machines can comprehend the world in a manner similar to humans. By leveraging the power of multiple modalities, these models open new possibilities in various fields, including healthcare, education, entertainment, and robotics. As research progresses and ethical considerations are taken into account, the potential of multimodal models continues to grow, heralding a future where technology can significantly enhance human life.
Multimodal AI is reshaping the landscape of artificial intelligence by offering more accurate, context-aware, and robust systems. Its applications span various domains, and the ongoing research promises to further enhance its capabilities and applications.
Training Challenges and Finding the Right Metrics in Multimodal AI
Training multimodal AI models and evaluating their performance involves navigating various challenges and finding the right metrics, which are crucial for ensuring the models' effectiveness and reliability.
Dataset Challenges in Multimodal AI
The foundation of a robust multimodal AI model is a diverse and high-quality dataset. However, several challenges arise in creating such datasets:
- Data Availability: Gathering enough data across multiple modalities is often difficult and expensive.
- Data Skew: A tendency for certain modalities to be overrepresented, leading to biased models.
- Annotation Costs: Labeling multimodal data accurately is time-consuming and requires expertise, making it a costly process.
To combat these challenges, solutions such as data augmentation, active learning, and transfer learning are employed. These methods help in increasing the size and diversity of datasets, reducing annotation costs, and leveraging pre-existing models to extract valuable features.
Training Challenges and Solutions
Training multimodal models involves:
- Balancing Modalities: Ensuring no single modality dominates due to more data or stronger features.
- Joint Representation Learning: Efficiently capturing relationships between different modalities, which can be computationally demanding.
- Evaluation Metrics: The need for specialized metrics tailored to the specific task of the model.
Solutions include the use of multimodal attention mechanisms, regularization techniques, and developing task-specific evaluation metrics. These approaches help in balancing the input from different modalities, preventing overfitting, and ensuring the model is accurately evaluated for its intended purpose.
Measuring Performance: Key Approaches and Metrics
The performance of Generative AI (GenAI), which includes many multimodal models, can be assessed through:
- Qualitative Evaluation: Human experts review and rate generated content for coherence, relevance, and fluency.
- Quantitative Evaluation: Using objective metrics like perplexity, BLEU score, and ROUGE score to assess model output.
Key metrics for evaluating GenAI performance include coherence and relevance, fluency, diversity, factual accuracy, bias and fairness, user satisfaction, and task completion and efficiency. These metrics help in assessing the logical flow, linguistic correctness, creativity, accuracy, and fairness of the generated content. They also gauge the model's effectiveness in completing specific tasks efficiently.
Challenges in Measuring Performance
Despite these approaches and metrics, several challenges persist in measuring GenAI performance:
- Lack of Ground Truth: Especially in creative tasks, establishing a definitive standard for evaluating outputs is difficult.
- Subjectivity: Many metrics rely on human judgment, introducing bias and variability.
- Multimodal AI Specific Challenges: Requires development of new metrics and techniques for evaluating models that operate across multiple domains.
- Real-world Complexity: Evaluating models in dynamic real-world scenarios adds another layer of complexity to performance measurement.
While challenges exist in dataset creation, training, and evaluation of multimodal AI models, by addressing these issues with effective strategies and metrics, we can enhance their reliability and usefulness in various applications. The ongoing development of new techniques and metrics, particularly for multimodal AI, is crucial to keep pace with the evolving complexities of these models.
Multimodal AI in Machine Learning: A New Era of Intelligent Systems
The advent of multimodal AI in machine learning marks a new era of intelligent systems, drastically enhancing how AI understands and interacts with the world. This shift towards multimodal AI involves integrating various types of data, such as text, video, images, sensor data, and audio, into a cohesive model. These systems process and interpret information from multiple sources, offering a more nuanced and comprehensive understanding of the world compared to unimodal AI systems, which are limited to a single data type.
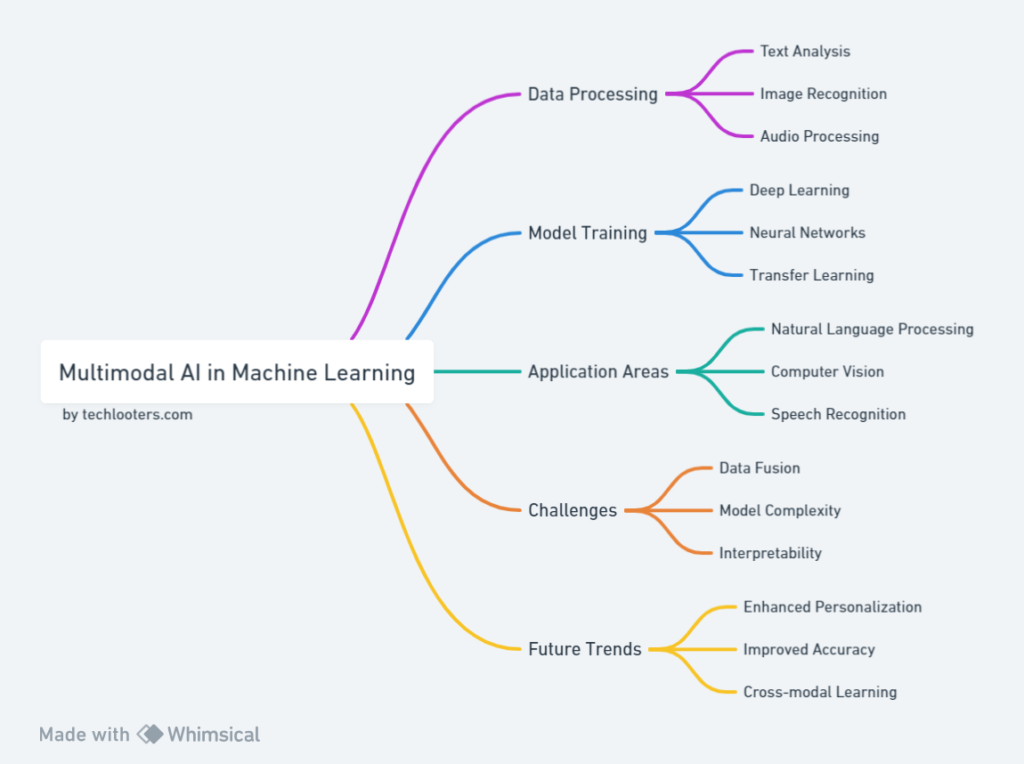
Unique Aspects of Multimodal AI
Multimodal AI is distinguished by its ability to fuse data from different modalities into a unified representation, often through complex neural network architectures like transformers. This fusion enables the models to capture intricate relationships and dependencies between various types of data, leading to a deeper comprehension of context. Such multimodal models are adept at generating responses, making predictions, and performing a variety of AI tasks with an unprecedented level of sophistication.
Benefits and Real-World Applications
The key advantages of multimodal AI include:
- Enhanced Understanding: By considering information from multiple sources, multimodal AI provides a richer context for analysis, leading to a more subtle understanding of data. This capability is especially beneficial in natural language processing, allowing for more accurate and relevant responses.
- Real-life Conversations: Multimodal models enable more natural interactions with AI assistants, as they can process both voice commands and visual cues like facial expressions and gestures, making the experience more personalized and engaging.
- Improved Accuracy: By integrating various modalities, multimodal AI models leverage the strengths of each to enhance accuracy and reduce errors. The advent of deep learning and neural networks has been pivotal in this regard, enabling the creation of complex, interconnected representations.
Challenges in Multimodal Machine Learning
Despite these advantages, multimodal AI poses several challenges:
- Fusion Mechanisms: Effectively merging information from different modalities is complex and requires selecting the right fusion method—early fusion, late fusion, or hybrid fusion—based on the specific task and data.
- Co-learning: Simultaneously training on varied modalities can lead to interference and catastrophic forgetting, where learning new tasks or modalities causes the model to forget how to perform previous tasks.
- Translation: Translating content that spans multiple modalities presents significant challenges in understanding the semantic content and relationships between different data types.
- Representation and Alignment: Creating effective multimodal representations and aligning different types of information accurately are critical yet challenging aspects of multimodal AI.
Multimodal AI in machine learning represents a transformative development, offering systems that can interact with the world in a more human-like manner. While there are challenges to be addressed, the potential of multimodal AI to revolutionize various fields and applications is immense, making it a key area of focus in the advancement of AI technology.
Real-World Examples of Multimodal AI in Action
Multimodal AI, characterized by its ability to process and integrate information from various sources such as text, images, audio, and video, is increasingly being applied across numerous sectors. These applications demonstrate the transformative potential of multimodal AI in understanding and interacting with the world more effectively.
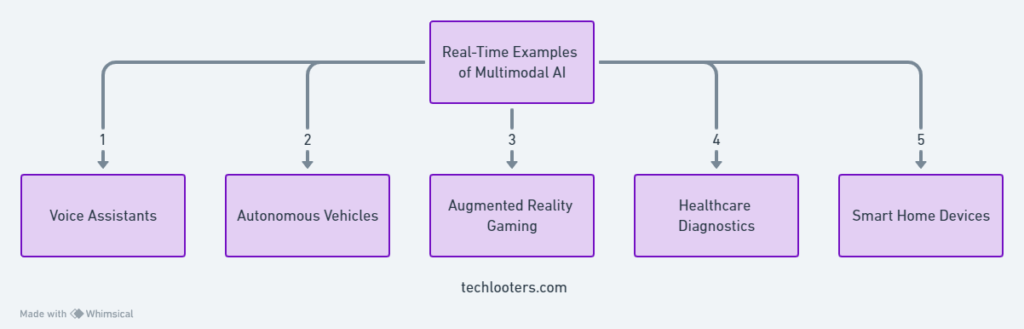
Image Captioning and Video Understanding
- Image Captioning: Multimodal models are instrumental in generating captions for images. They analyze the visual features and context to produce accurate and descriptive captions. This technology finds applications in image retrieval, aiding visually impaired individuals, and inspiring creative writing.
- Video Understanding: By processing both audio and visual information, multimodal models have revolutionized video analysis. Applications include automatic video summarization, real-time subtitles for accessibility, and surveillance video analysis for safety and security.
Speech-to-Text with Visual Context
Enhanced Speech Recognition: Multimodal models incorporate visual information from the speaker’s lips and facial expressions to improve speech recognition, particularly in noisy environments or with heavy accents. This technology facilitates effortless transcription, improves communication for individuals with speech impairments, and creates personalized educational tools.
E-commerce, Healthcare, and Entertainment
- E-commerce: In the e-commerce sector, multimodal models merge textual reviews with product images to offer a richer understanding of products, enhancing customer experience and satisfaction.
- Healthcare: Multimodal AI is increasingly used in healthcare for integrating medical images with patient notes, leading to more comprehensive diagnoses and improved patient care.
- Entertainment: Customizing content based on both textual and visual user preferences is another area where multimodal AI is making an impact, offering more personalized and engaging entertainment experiences.
These examples highlight the versatility and effectiveness of multimodal AI in various real-world applications. From improving accessibility and enhancing user experience to advancing healthcare diagnostics and revolutionizing entertainment, the applications of multimodal AI demonstrate its potential to significantly impact and benefit various aspects of daily life and professional domains.
Top 5 Multimodal AI Tools Transforming Industries
- Google Gemini: A multimodal Language Model (LLM) that can identify and generate text, images, video, code, and audio. It comes in three versions: Gemini Ultra, Gemini Pro, and Gemini Nano, each designed for specific user needs. Gemini Ultra, in particular, has shown exceptional performance, surpassing GPT-4 on most benchmarks.
- ChatGPT (GPT-4V): Powered by GPT-4 with vision, this tool introduces multimodality by allowing inputs of text and images. It supports a mix of text, voice, and images in prompts and can respond in various AI-generated voices. This tool has gained significant traction, with a vast user base.
- Inworld AI: This character engine enables developers to create non-playable characters (NPCs) and virtual personalities for digital worlds. By leveraging multimodal AI, Inworld AI allows NPCs to communicate through natural language, voice, animations, and emotions, significantly enhancing digital experiences.
- Meta ImageBind: An open-source multimodal AI model that processes text, audio, visual, movement, thermal, and depth data. It's notably the first AI model capable of combining information across six modalities, used creatively for merging disparate inputs like audio and images.
- Runway Gen-2: Specializing in video generation, Runway Gen-2 accepts text, image, or video input to create original video content. It offers functionalities like text-to-video, image-to-video, and video-to-video, allowing users to replicate styles, edit content, and achieve high-fidelity results, making it ideal for creative applications.
These tools are at the forefront of the multimodal AI revolution, showcasing the potential of combining various modalities to enhance user experiences, creative processes, and industry applications.
Frequently Asked Questions (FAQs) about Multimodal AI
What is Multimodal AI?
Multimodal AI refers to artificial intelligence systems that can process and understand multiple types of data or 'modalities', such as text, images, audio, and video, simultaneously.
How Does Microsoft Use Multimodal AI?
Microsoft employs multimodal AI in various applications, including enhancing AI capabilities in productivity tools, healthcare, and creative industries, focusing on responsible AI development.
What are Multimodal AI Models?
Multimodal AI models are AI systems designed to integrate and interpret data from different modalities, like text and images, for a more comprehensive understanding and analysis.
What are the Applications of Multimodal AI?
Applications include image captioning, video analysis, speech-to-text with visual context, enhanced e-commerce experiences, and advancements in healthcare diagnostics.
What is the Meaning of Multimodal AI?
The meaning of multimodal AI lies in its ability to process and synthesize information from various sources, leading to a more nuanced and intelligent AI system.
Can You Provide Examples of Multimodal AI?
Examples include Google Gemini for generating various types of content, ChatGPT (GPT-4V) for text and image processing, and Meta ImageBind for artistic creations using multiple data types.
What are the Top 5 Multimodal AI Tools?
Top tools include Google Gemini, ChatGPT (GPT-4V), Inworld AI, Meta ImageBind, and Runway Gen-2, each offering unique capabilities in processing and generating multimodal content.
How is Multimodal AI Transforming Machine Learning?
Multimodal AI is transforming machine learning by enabling models to understand complex and varied data types, leading to more intelligent, accurate, and adaptable AI systems.
I just could not depart your web site prior to suggesting that I really loved the usual info an individual supply in your visitors? Is gonna be back regularly to check up on new posts.